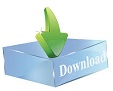

and Mohamed S., “ Variational Inference with Normalizing Flows,” International Conference on Machine Learning (ICML), 2015, pp. and Bengio Y., “ Generative Adversarial Nets,” Advances in Neural Information Processing Systems (NeurIPS), Curran Associates, Inc., Red Hook, NY, 2014, pp. and Welling M., “ Auto-Encoding Variational Bayes,” arXiv: 1312.6114, 2013. and Welling M., “ An Introduction to Variational Autoencoders,” arXiv:1906.02691, 2019. and Solenthaler B., “ Deep Fluids: A Generative Network for Parameterized Fluid Simulations,” Computer Graphics Forum, Vol. 38, No. 2, 2019, pp. 59–70. and Thuerey N., “ Latent Space Physics: Towards Learning the Temporal Evolution of Fluid Flow,” Computer Graphics Forum, Vol. 38, No. 2, 2019, pp. 71–82. D., “ Recovering Missing CFD Data for High-Order Discretizations Using Deep Neural Networks and Dynamics Learning,” Journal of Computational Physics, Vol. 395, Oct. L., “ Deep Learning for Universal Linear Embeddings of Nonlinear Dynamics,” Nature Communications, Vol. 9, No. 1, 2018, Paper 4950. J., “ Deep Dynamical Modeling and Control of Unsteady Fluid Flows,” Advances in Neural Information Processing Systems (NeurIPS), Curran Associates, Inc., Red Hook, NY, 2018, pp. and Jayaraman B., “ Deep Multilayer Convolution Frameworks for Data-Driven Learning of Fluid Flow Dynamics,” 2018 Fluid Dynamics Conference, AIAA Paper 2018-3091, 2018. W., “ Linearly Recurrent Autoencoder Networks for Learning Dynamics,” SIAM Journal on Applied Dynamical Systems, Vol. 18, No. 1, 2019, pp. 558–593.
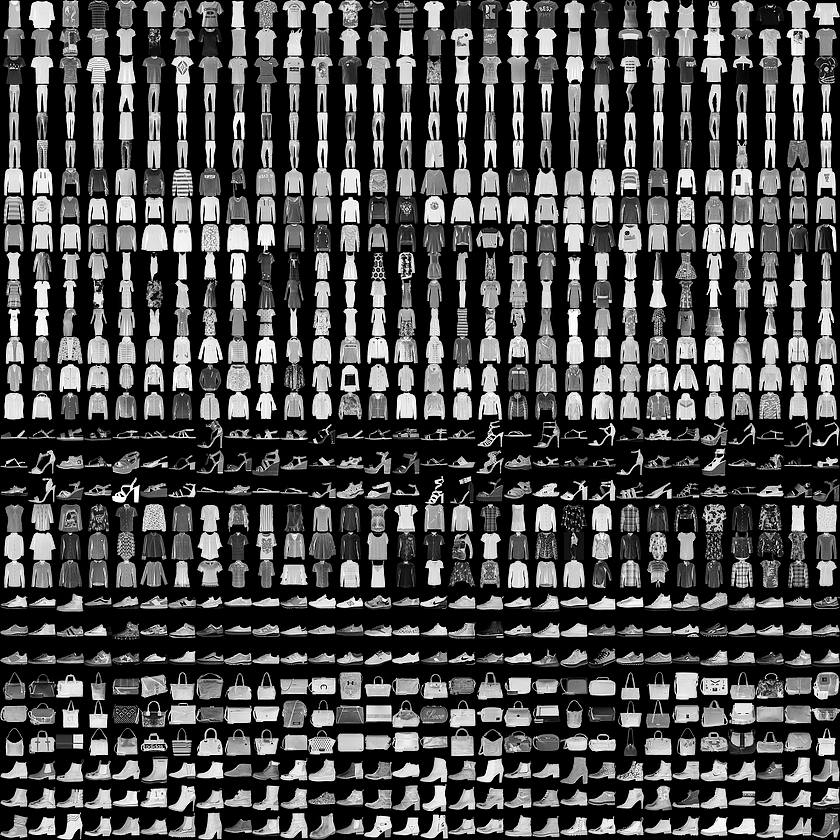
for Industrial and Applied Mathematics, Philadelphia, PA, 2016, pp. and Proctor J., Dynamic Mode Decomposition: Data-Driven Modeling of Complex Systems, Soc. N., “ On Dynamic Mode Decomposition: Theory and Applications,” Journal of Computational Dynamics, Vol. 1, No. 2, 2014, pp. 391–421. and Farhat C., “ Neural Networks Predict Fluid Dynamics Solutions from Tiny Datasets,” arXiv:1902.00091, 2019. and Carlberg K., “ Deep Conservation: A Latent Dynamics Model for Exact Satisfaction of Physical Conservation Laws,” arXiv:1909.09754, 2019. T., “ Model Reduction of Dynamical Systems on Nonlinear Manifolds Using Deep Convolutional Autoencoders,” Journal of Computational Physics, Vol. 404, March 2020, Paper 108973. and Farhat C., “ Efficient Non-Linear Model Reduction via a Least-Squares Petrov–Galerkin Projection and Compressive Tensor Approximations,” International Journal for Numerical Methods in Engineering, Vol. 86, No. 2, 2011, pp. 155–181. and Peraire J., “ Balanced Model Reduction via the Proper Orthogonal Decomposition,” AIAA Journal, Vol. 40, No. 11, 2002, pp. 2323–2330. J., “ Recent Advances in Surrogate-Based Optimization,” Progress in Aerospace Sciences, Vol. 45, Nos. 1–3, 2009, pp. 50–79. K., “ Surrogate-Based Analysis and Optimization,” Progress in Aerospace Sciences, Vol. 41, No. 1, 2005, pp. 1–28. I hope this helps you, as I saw you had inquired in 2010 and again in 2018.Sacks J. Step 4: Select the desired import variable from the drop-down box located in the second toolbar in Paraview Step 2: Before clicking the green 'apply' button, you must select the desired import variables from the 'Cell Arrays' and/or 'Point Arrays' boxes. Step 4: Select 'Ensight Gold file (*.case)' as the file type Step 3: Make sure the drop-down box says 'export mesh and solution data'. You will see them being tallied in the field below this box. You must click on each tab and highlight the desired export variables. Step 2: In the new save window, there is a box on the right with a number of tabs (~5). In paraview its possible to view the grid, but no velocity information is available. Afterwards I open the file with paraview. As recommended i export my file from star ccm as a. Post process star ccm+ data with paraview.
#MNIST FOR PARAVIEW HOW TO#
How to see a Star CCM+ Simulation with Paraview? - CFD Online Discussion Forums
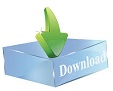